Case Studies
Case Study — CareATC: Breaking the Rules
With a mission to help employers save money on healthcare by improving their members’ health, CareATC was the first in its sector to implement rules-based predictive analytics for member costs and health risks at an individual level.
With a mission to help employers save money on healthcare by improving their members’ health, CareATC was the first in its sector to implement rules-based predictive analytics for member costs and health risks at an individual level. To further improve the predictive accuracy and the efficiency of its outreach programs, CareATC decided to explore artificial intelligence/machine learning (AI/ML) as an alternative to rules-based risk stratification.
Impact Summary
- 75% of individuals for whom ClosedLoop’s predicted costs were more accurate than a rules-based system
- 30% of unplanned hospital admissions correctly predicted by ClosedLoop in the top 5% by risk
- 2.3X increase in identification of unplanned hospital admissions vs. baseline
Read the case study to learn more.
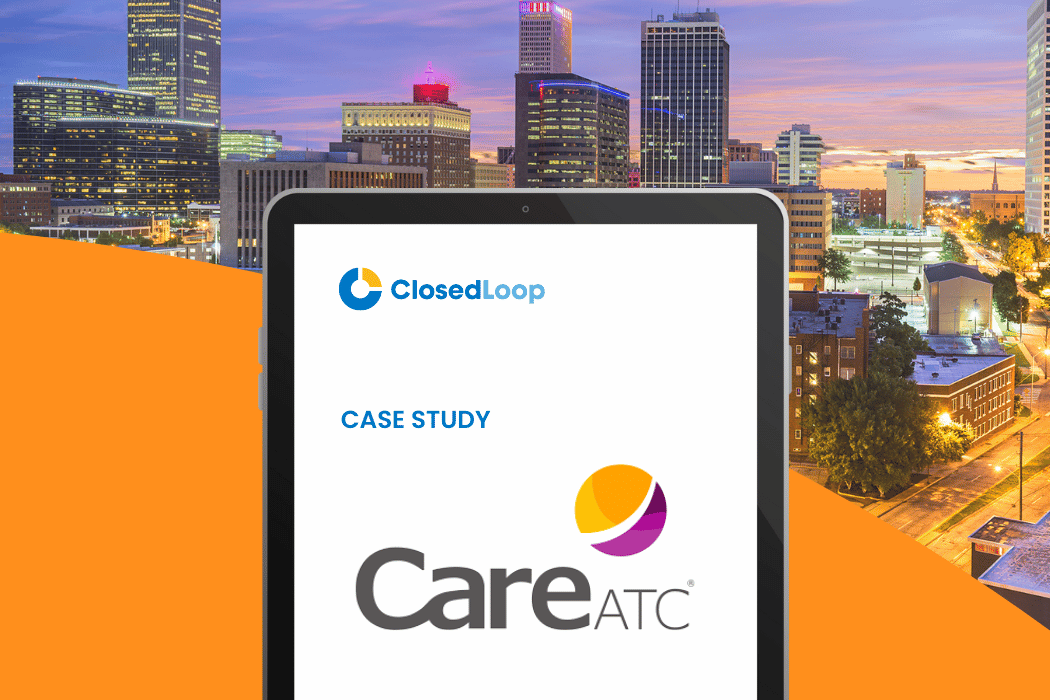
Read the case study
Related Resources
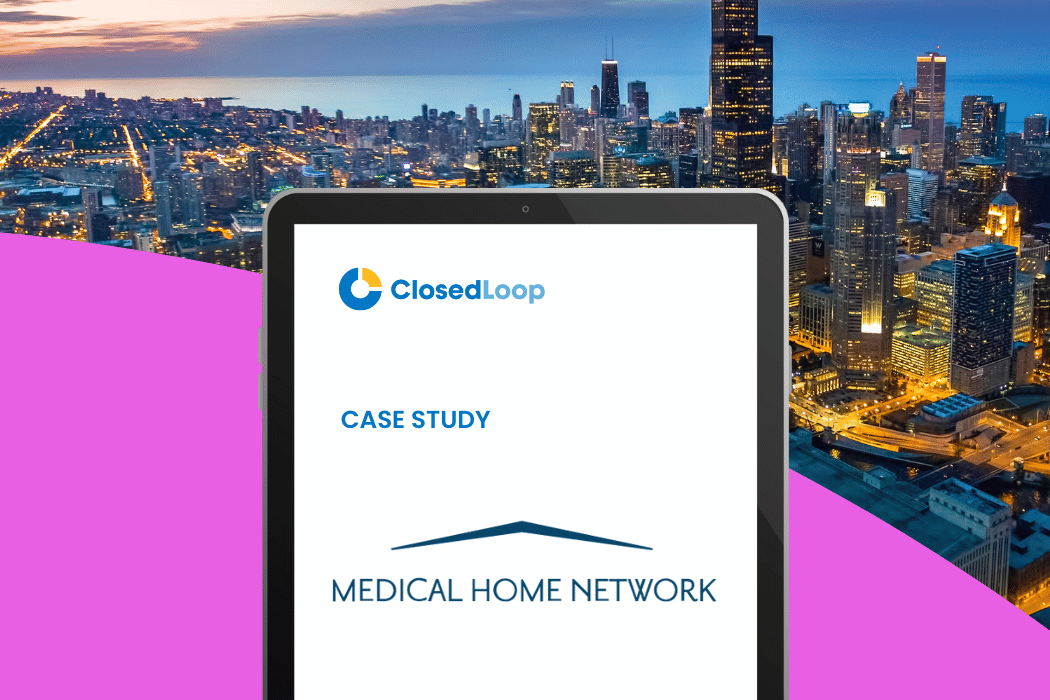
Case Study — Medical Home Network Enriches Predictions with SDoH Data
Read how incorporating SDoH data into its models allowed Medical Home Network to proactively identify and connect individuals likely to incur high costs with care management resources and community-based organizations.
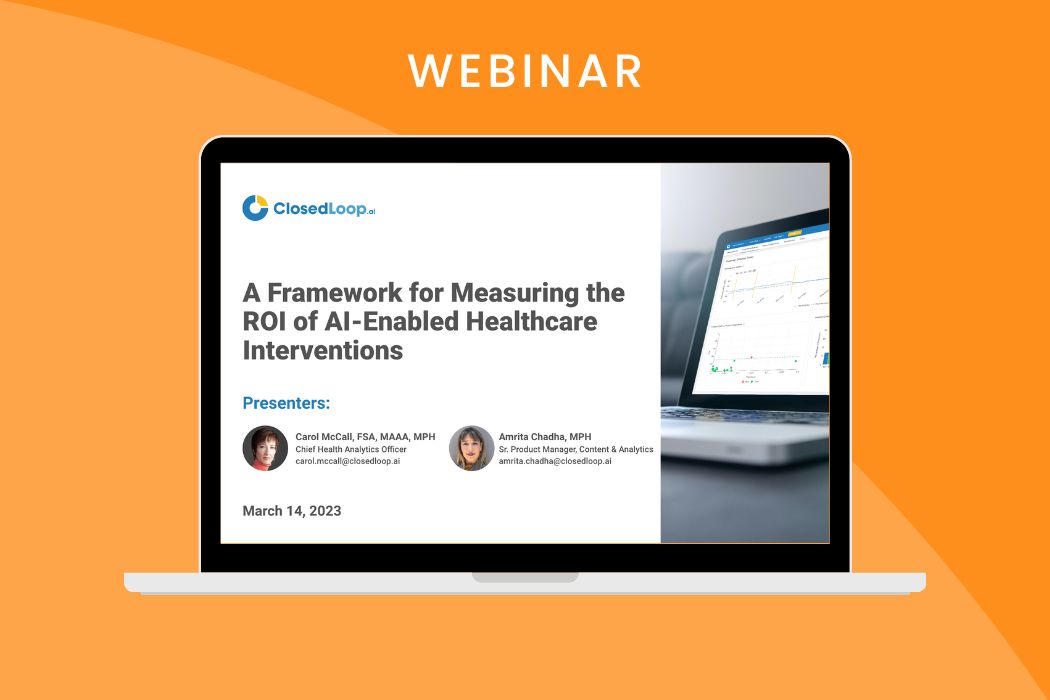
A Framework for Measuring the ROI and Health Equity Impact of AI-Enabled Health Programs
Discover a useful framework that your organization can use to evaluate programs’ ROI and impact on health equity, especially when introducing an artificial intelligence / machine learning component.
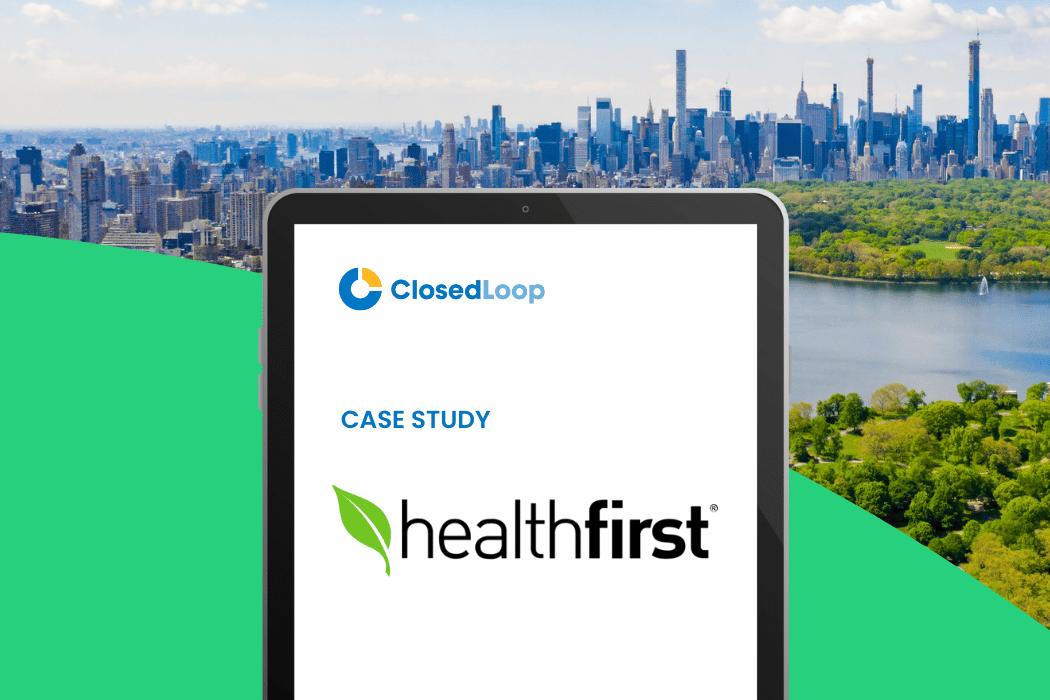
Case Study — Healthfirst Achieves Agile AI/ML in Healthcare
Learn how Healthfirst’s analytics team has dramatically enhanced its ability to train, test, and deploy AI-based models. The team has developed 978 custom features to supplement 612 features created using ClosedLoop’s pre-built templates.