White Papers
Where Most Healthcare AI/ML Deployments Go Wrong
Read the white paper to explore three of the most common ways healthcare AI/ML models go wrong, and how you can ensure they go well.
More than ever before, artificial intelligence/machine learning (AI/ML) models have the potential to improve healthcare and decision-making; and the stakes are high. Actions taken or not taken based on predictions will have an impact on people’s health. Systemwide decision-making informed by tools working at a suboptimal level can result in missed opportunities to improve health outcomes, or even exacerbate health disparities. Considering what’s at stake, can data scientists accept a predictive model deployment rate of only 1 in 10?
Let’s explore three of the most common ways healthcare AI/ML models go wrong, and how you can ensure they go well.
- Data Quality
- Shifts in Underlying Data
- Ever-Changing Healthcare Terminologies
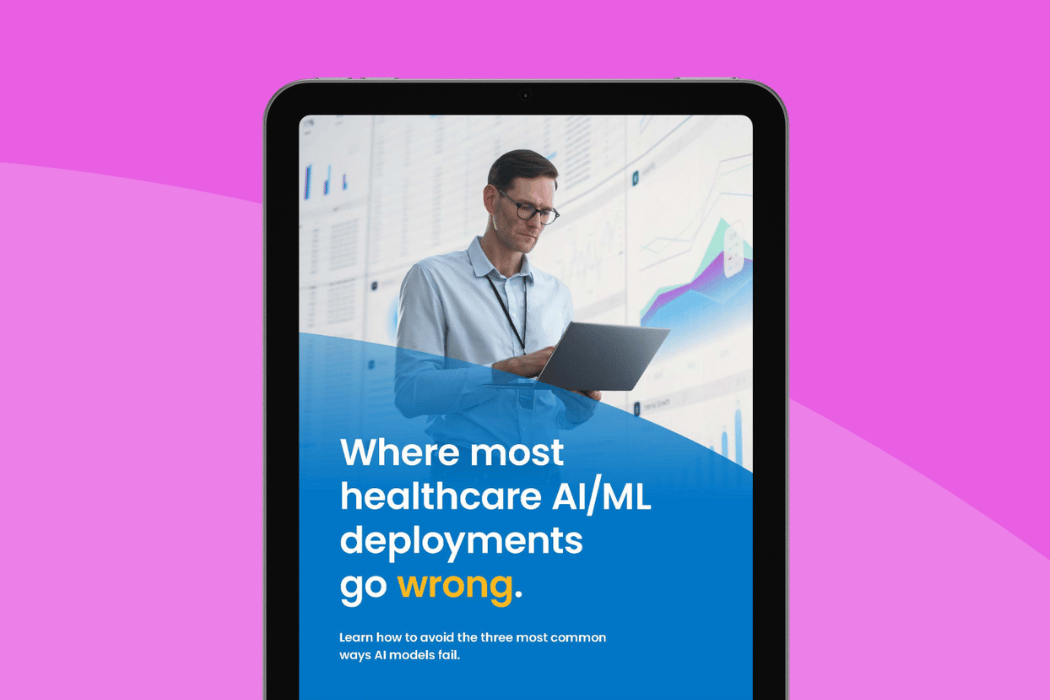
Read the white paper
Related Resources
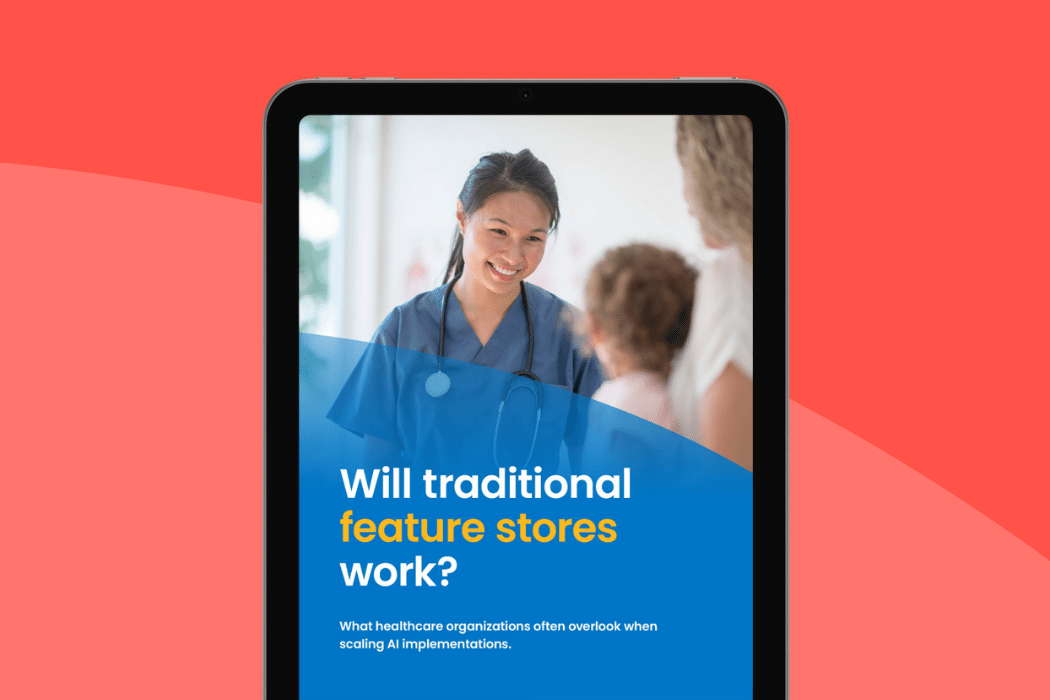
Machine Learning in Healthcare: Will Traditional Feature Stores Work?
Read the white paper to learn how a healthcare feature store can accelerate time-to-value.
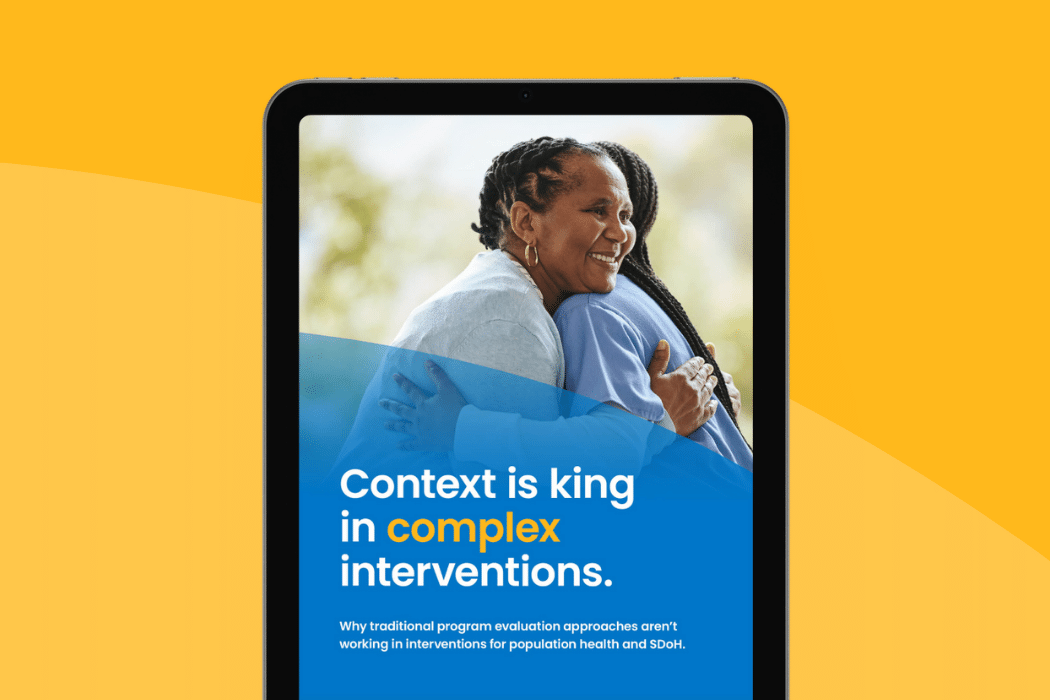
Context is King in Complex Interventions
Traditional program evaluation approaches fall short when it comes to assessing complex interventions, but HCOs must be capable of evaluating whether or not their programs work if they are to succeed in healthcare’s new business model. Without a “gold standard” evaluation approach, how can HCOs measure the sustainability and impact of their interventions?
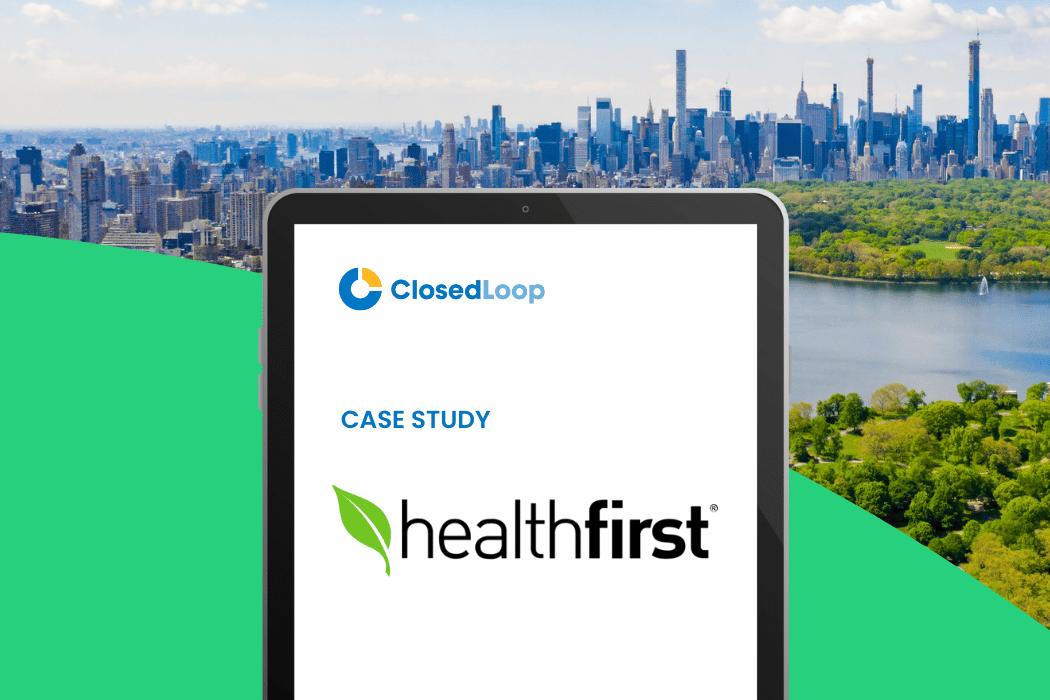
Case Study — Healthfirst Achieves Agile AI/ML in Healthcare
Learn how Healthfirst’s analytics team has dramatically enhanced its ability to train, test, and deploy AI-based models. The team has developed 978 custom features to supplement 612 features created using ClosedLoop’s pre-built templates.